OpenWeb, with Jigsaw’s Perspective API, releases data demonstrating the ability of technology to positively affect the quality of online conversations. These nudge theory examples study involved nearly half a million comments and 50k users across sites including AOL, Salon, Newsweek, RT, and Sky Sports.
As a company on a mission to elevate conversations online, we want to inspire an open exchange of ideas across the web — with less hostility and toxicity. We do this by building innovative conversation technologies and designing experiences that bring people together. We believe (most) people are inherently good — they understand right from wrong. With the right encouragement, we can not only inform individual user behavior but also impact overall community health in a positive way.
Fundamentally encouraging good behavior is equally as important as removing the bad actors to support a thriving community. Our robust moderation platform built with multiple layers of protection, powered by AI/ML, trusted API integrations such as Jigsaw’s Perspective API, and a proprietary moderation algorithm identifies and removes behaviors that put the publisher and community at risk. In addition, our user-centric products expose high-quality content and incentivize users to create healthier, engaging content. Our goal is to encourage healthy debate and make room for diverse opinions, without suppressing free speech.
We use Jigsaw’s Perspective API to advance our mission to improve online conversations through innovative technologies. Perspective API is a tool developed by Jigsaw that uses machine learning to identify and predict whether a comment is abusive and/or toxic to simplify the moderation process. After successfully employing their API within our moderation technology for a few years, we decided to experiment with a new feature, in collaboration with Jigsaw.
Real-time Feedback
At OpenWeb, we run Perspective ML models on millions of comments to catch abuse and toxicity in real-time. Combining this scoring mechanism with our proprietary technology, we now offer a Real-time Feedback feature, that gives users an opportunity to change their message, if it is suspected to break the Community Guidelines. The feature uses the nudge concept, a known theory in behavioral sciences that proposes positive reinforcement and indirect suggestions as ways to influence the behavior and decision making of groups or individuals. Here are some nudge theory examples:
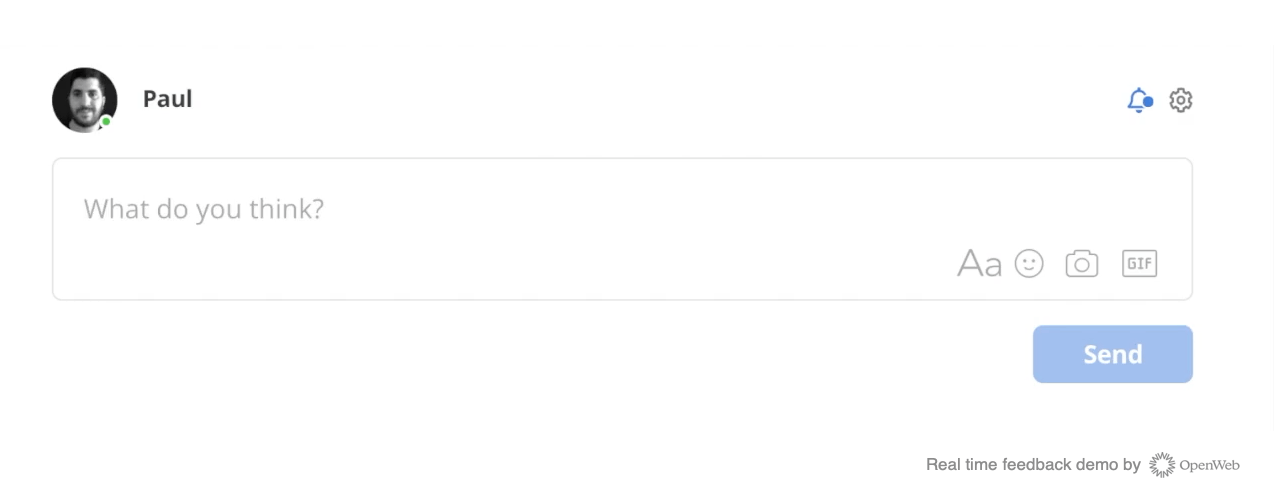
Every time a user submits a comment, it goes through the moderation algorithm. If the system identifies the content as risky/offensive or is misaligned with the publisher’s Community Guidelines instead of rejecting it automatically or sending for additional human review, a message is presented to the user urging them to take another look at what they wrote. The user can either choose to edit their comment and repost or they can post it anyway, accepting the outcome. Users get one chance per comment to rethink their message before it is submitted, in order to deter them from gaming the system.
We set out to run this feature as an experiment of nudge theory examples with the following hypotheses:
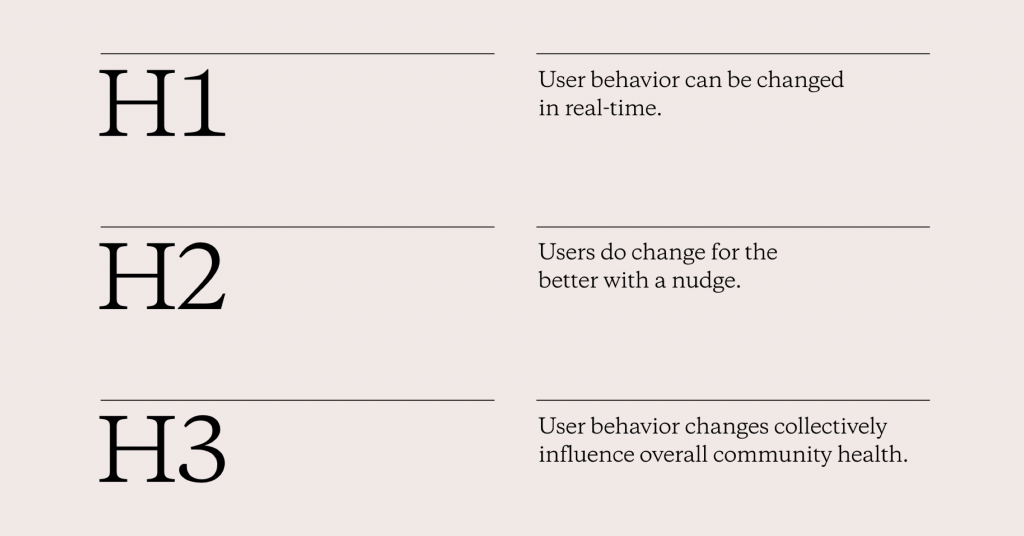
Experiment
We set up the experiment on a few of our top publishers including AOL, Salon, Newsweek, RT, and more, to test if the above hypotheses can be validated with data. We ensured the partners we chose represented a diverse group of audiences, interests, engagement levels, and industry verticals managing any selection bias affecting the experiment.
The Real-time Feedback was released starting May 2020 and examined over 50k users and 400k comments. Two test groups were set up using 50% of publisher traffic as the base.
- Group A / test group: Users that received the feedback
- Group B / control group: Users that didn’t receive the feedback
As feedback messages have the potential to evoke different responses, we leveraged this opportunity to test a variety of messages and measure conversion. For each initial moderation decision (rejected automatically or required review) we presented two versions of the message — one with positive motivation versus another with direct negative feedback. The messages were optimized over time based on user responses.
As expected, different nudges elicited different behaviors. Clearly expressed feedback and call-to-action increased the probability of users taking action by 10%-20%.
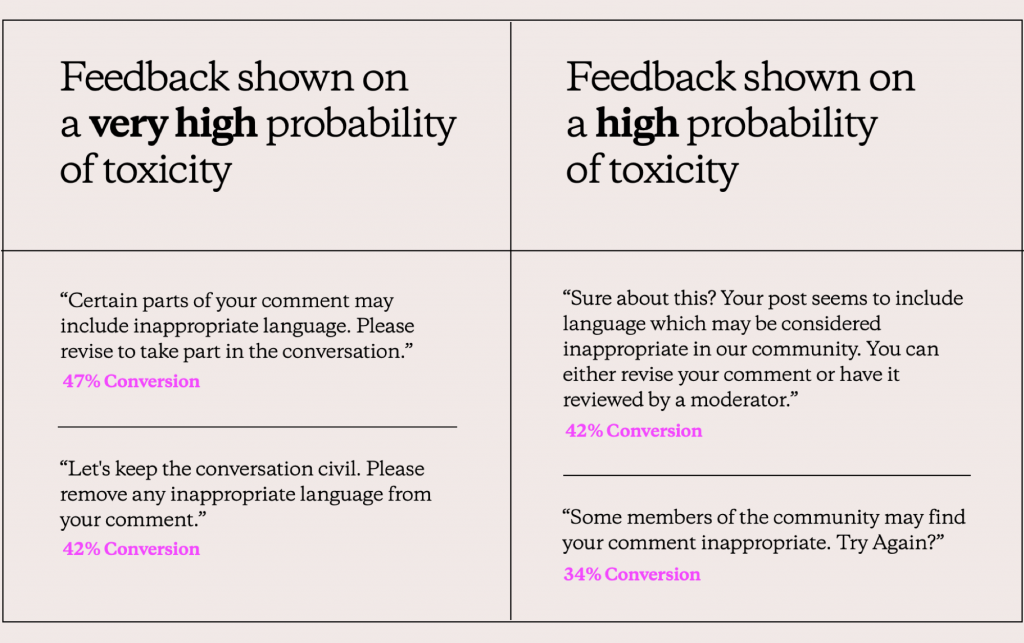
Results
We analyzed these nudge theory examples and results under three lenses:
- Feature funnel – How did the user navigate the nudge/feedback experience?
- User behavior – How did the feature impact individual user interactions?
- Community health – How did the feature impact the community as a whole?
Feature Funnel
H1. User behavior can be changed in real-time.
34% of users edited their comment upon nudge.
We identified 4 key paths users could take with this feature:
- Edit the comment – 34% of users edited their comment upon nudge
- Post the comment without edits – 36% of users posted the comment anyway
- Abandon the effort – 12% of users left without trying to edit or comment further
- Repost – 18% of users test the feature by reposting either by refreshing the page or as a reply to an existing comment
From 34% of users who chose to edit their comment, 54% changed it to be immediately permissible ie. those messages were either immediately published or were sent to a moderator review instead of being automatically rejected.
Edit Behavior
In order to understand how users edit their comments, we turned to qualitative analysis by manually coding the comments. We analyzed the actual content before receiving the feedback and after editing the comment. Hundreds of randomly sampled edited comments were categorized into 7 different types of behaviors:
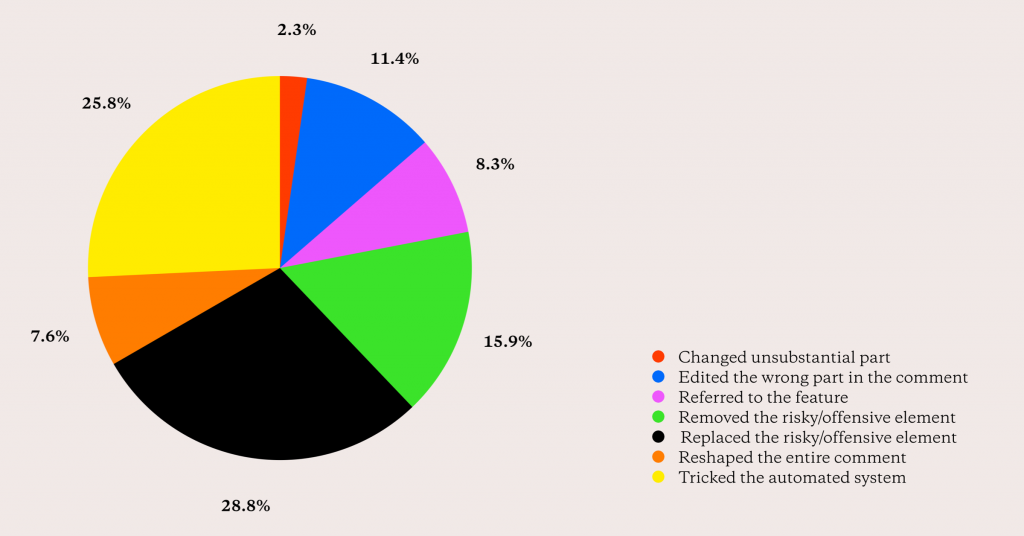
Before: “Nice try. China will simply print “Made in USA, Germany, UK, France”, they’ll ship their crap through other countries. You can’t stop China with regulations. Dems of course will call you a racist.
After: “Nice try. China will simply print “Made in USA, Germany, UK, France”, they’ll ship their stuff through other countries. You can’t stop China with regulations.”
2. Removed the risky/offensive element: Users understood what’s wrong with their comments and decided to completely remove the risky/offensive element.
Before: “obama is a pos liar and that goes for the rest of them too.”
After: “obama is a liar and that goes for the rest of them too.”
3. Reshaped the entire comment: Users decided to erase their original comment and express themselves in a new way.
Before: “Screw you, you low life scum sucking bitch.”
After: “Waters you’re headed to hell!!”
4. Tricked the automated system: Users understood what’s wrong and decided to find creative ways to deliver their message.
Before: “The Bstrd will never be touched!”
After: “The b s t r d will never be touched!”
5. Referred to the feature: Users decided to change their first version and share their thoughts on the feedback they were given.
Before: “Typical Demo-rat! When they do not have a cognizant, legitimate argument they break out the race card! They have done that so often it not only becomes meaningless, it verifies the object of the charge is correct!”
After: “What is offensive about the truth and what I said? Take your censor-ship and stuff it!”
6. Edited the wrong part in the comment: Users that probably didn’t understand what’s wrong with their content and didn’t change the risky/offensive element.
User Behavior
H2: Users do change for the better with a nudge.
Overall 12.5% lift in civil and thoughtful comments being published.
The results at the user behavior level are fascinating. Though we need to continue analyzing to see if the behavior changes are long-term, the substantial immediate impact proves the effectiveness of moderation and quality tools. We measured success with the following key metrics:
Approval rate: Measures the health of the user by the number of comments that were approved, divided by the number of total comments submitted. The results showed that the approval rate for users from Group A (the test group that received the feedback) were 2%-4% higher compared to users from Group B during the experiment.
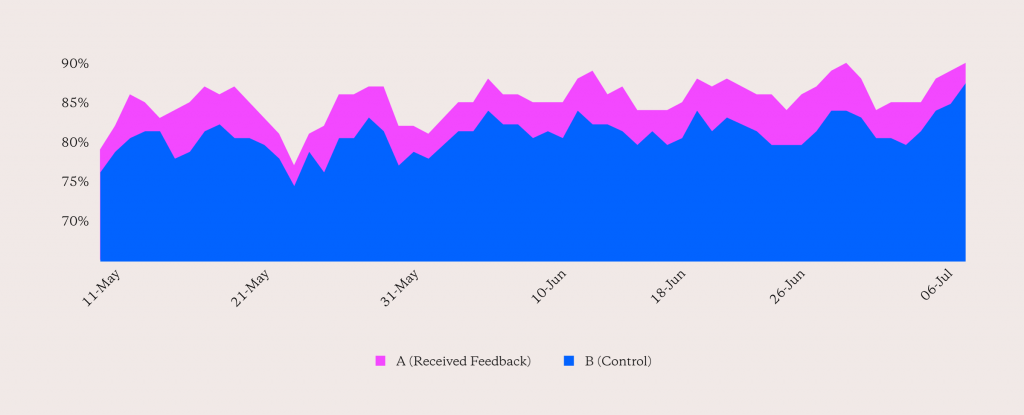
Retention rate: Measures the likelihood of users returning over time. Group A user’s retention rate was on average 3.25% higher with a cumulative increase of 26% compared to Group B’s during the nudge theory examples experiment.
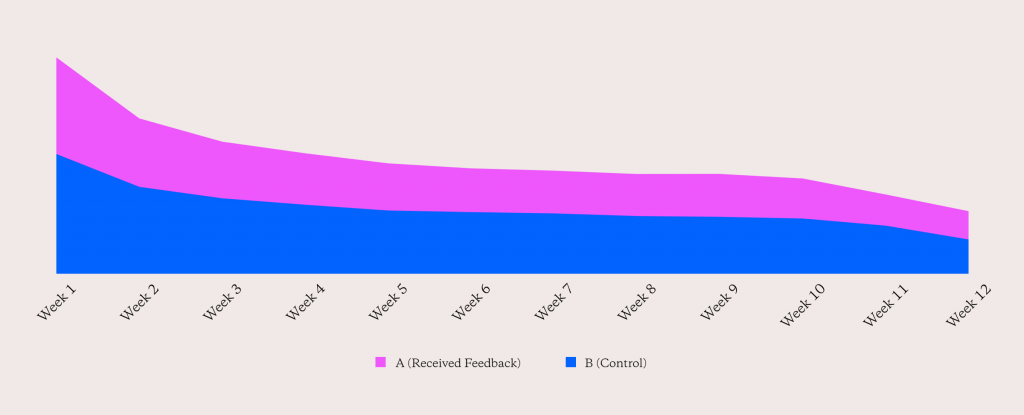
Engagement rate: Measures the activity rate of the users by dividing the number of active users over the total users. It is crucial to ensure that there is no long-term negative impact on the users who received the feature. The results showed that Group A’s engagement rate was slightly higher at 0.6% compared to Group B.
Community Health
H3: User behavior changes collectively influence overall community health.
Community approval rate increased by 2.5%-4.5%
Community safety rate increased by 5%-6%
The increased engagement and retention rate at the individual level invariably leads to more active users and thriving communities on publishers’ sites. We reviewed the same key metrics at the community level, to assess how individual actions contributed to aggregated health and engagement levels of the overall community. It is important that we ensure overall community engagement is not negatively impacted by this new feature. We did not notice a lift in the engagement rate, but we saw a significant increase in the overall quality of conversations.
*Approval rate is the percentage of approved comments out of total comments. Safety is the percentage of good comments exposed out of the total comments exposed.
Nudge Theory Examples in Conclusion
The overall positive outcome of this experiment reinforces our belief that quality spaces and civility ques drive a better, healthier conversation experience. A gentle nudge can steer the conversation in the right direction and provide an opportunity for users with good intentions to participate. The feature provides more transparency and education throughout the user engagement journey boosting loyalty and overall community health.
But when it comes to moderation technologies there is no one size fits all. There will always be a certain percentage of users who will try to break the rules and circumvent the system. We believe this data analysis has also helped us understand and detect online trolls faster and better. If a user is repeatedly ignoring nudges and trying to trick the system, it warrants stronger tools such as auto suspension. OpenWeb allows publishers to suspend a user from participating in the conversation if they attempt to post a rejected comment multiple times.
At OpenWeb we are continually improving our moderation decision-making and tools. We want to reduce false negatives, train our algorithms to detect online incivility better and reward users who change positively. Let’s break the cycle of negativity and hostility online, which does not necessarily mean censorship. We want open forums where everyone’s voice matters, where people assume the best intentions and everyone has a place to participate on the open web.